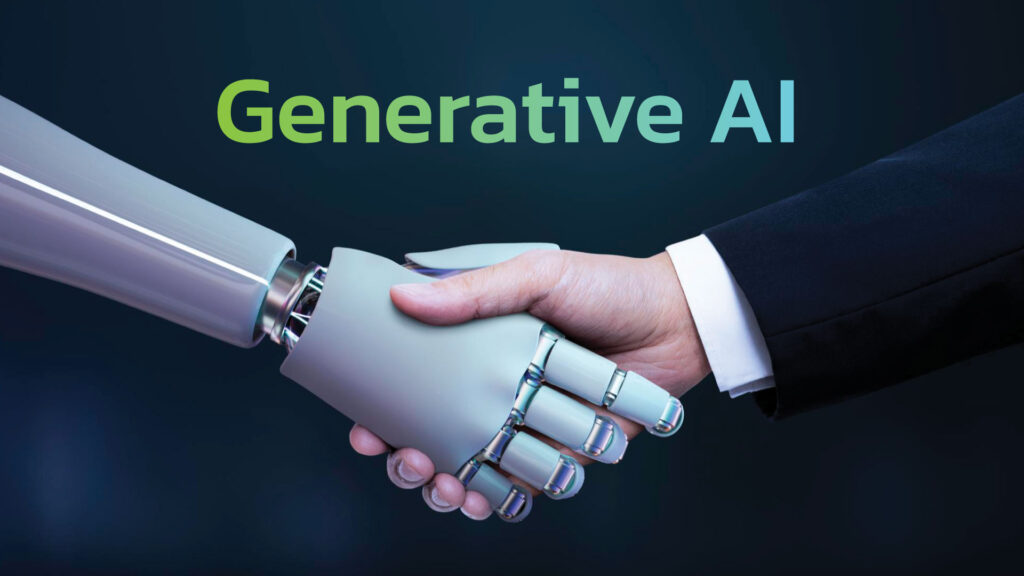
Introduction to Generative AI
Generative AI is revolutionizing the landscape of artificial intelligence by enabling machines to create content, from images and text to music and code. This transformative technology is not only advancing digital creativity but also reshaping how industries operate. As a subset of artificial intelligence, generative AI uses complex machine learning algorithms to generate new data that mimics existing content. From art and entertainment to business and healthcare, the applications are expanding rapidly.
What is Generative AI?
Generative AI refers to systems that can generate text, images, sounds, and other types of data. It is primarily powered by deep learning models, such as Generative Adversarial Networks (GANs) and Variational Autoencoders (VAEs). These models learn patterns and structures from large datasets and use that knowledge to produce new, similar content.
Unlike traditional AI, which typically follows a set of predefined rules, generative AI leverages AI modeling techniques to innovate. This shift from rule-based AI to learning-based AI is enabling more human-like and creative outputs. For example, an AI images generator can create realistic pictures of people who do not exist, while a language model can write poetry, essays, or even software code.
How Generative AI Works
At the heart of generative AI are machine learning algorithms that process and learn from vast amounts of data. These algorithms are trained to understand the features and nuances of the input data. Once trained, they can generate new data points that resemble the training set but are entirely original.
A typical example is the use of GANs, which consist of two neural networks: a generator and a discriminator. The generator creates new data samples, while the discriminator evaluates them against real data. Through continuous iteration, the generator becomes adept at producing highly realistic outputs.
AI modeling plays a crucial role here. It involves designing and training neural networks that can recognize intricate data patterns. The better the modeling, the more coherent and sophisticated the AI-generated content.
Applications of Generative AI
The potential applications of generative AI are vast and growing. In the creative industries, it is used for generating music, art, and even screenplays. Filmmakers can use it to de-age actors or simulate visual effects. Writers use it for brainstorming ideas and enhancing narratives.
In business, generative AI aids in creating marketing content, generating product designs, and automating customer service responses. Retailers use AI images generators to create visual content for advertising without needing expensive photoshoots.
Healthcare is also benefiting from generative AI. It is being used to model new drugs, generate synthetic medical images for training, and assist in diagnosis by analyzing medical data in innovative ways.
Challenges and Ethical Considerations
Despite its promise, generative AI comes with challenges and ethical dilemmas. One major concern is the creation of deepfakes—hyper-realistic fake images or videos that can spread misinformation. There are also issues related to copyright, data privacy, and the potential for job displacement.
Transparency in how AI models are trained and used is critical. Users need to understand whether content is human-made or machine-generated. Ensuring accountability and developing regulatory frameworks will be essential as this technology becomes more prevalent.
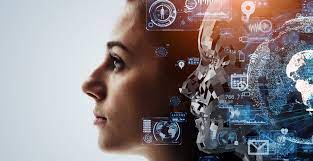
The Future of Generative AI
Generative AI is poised to become a cornerstone of future technology. As AI modeling becomes more advanced and machine learning algorithms more efficient, the quality and variety of generative outputs will continue to improve.
We can expect more personalized and interactive applications, such as virtual assistants that create custom content in real-time or AI artists that adapt to individual tastes. Education, entertainment, and enterprise solutions will all be enriched by generative AI.
Moreover, open-source platforms and AI-as-a-service models are making generative tools accessible to everyone, democratizing creativity and innovation. The key will be balancing innovation with responsibility.
Conclusion
Generative AI stands at the frontier of technological advancement, blending the power of machine learning algorithms with human creativity. From generating realistic visuals with AI images generators to crafting narratives with language models, its influence is growing across sectors. By harnessing AI modeling and ethical practices, generative AI can unlock new realms of possibility while ensuring its impact remains positive and inclusive.